Quantum vs. AI for Drug Discovery
AI hype vs. reality
In recent years, Artificial Intelligence (AI) has enabled significant strides in drug discovery, utilizing machine learning algorithms to predict molecular interactions and optimize drug candidates.
However, AI solutions come with their own limitations.
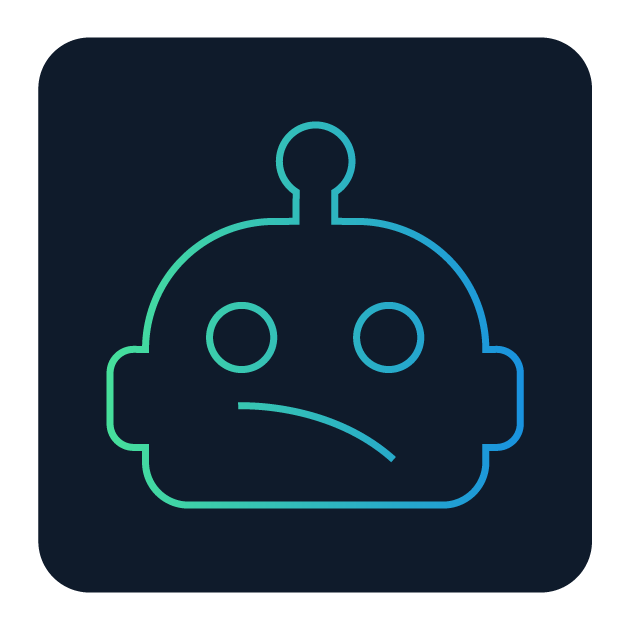
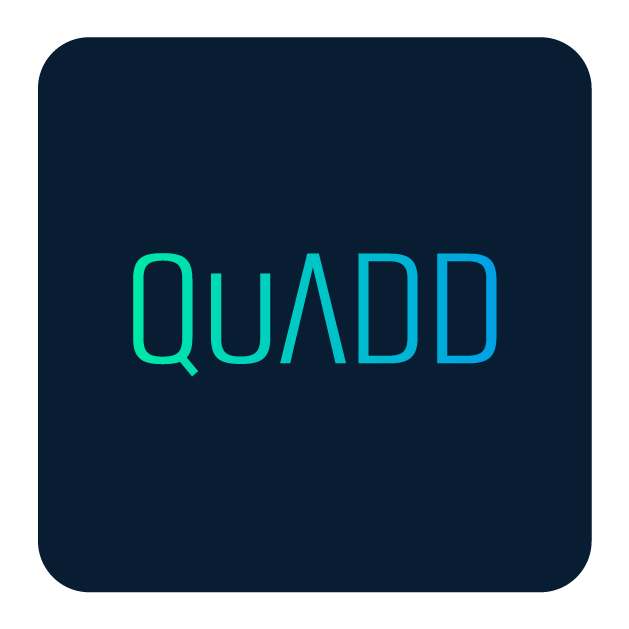
Data limitations diminish AI applicability and diversity
AI relies heavily on large datasets to train its models. This limitation restricts its applicability to drug targets that have sufficient and relevant training data, leaving out novel or less-studied targets.
Quantum-Aided Drug Design (QuADD) is not bound by the constraints of training data. Using physics-based calculations with no need for parametrization, it can be confidently applied to any drug target with a known 3D structure.
This broad applicability is crucial for identifying and optimizing drug candidates for a wider range of targets, including those that are novel or have limited existing data.
Black box nature undermines explainability and confidence
One of the significant drawbacks of AI in drug discovery is its ‘black box’ nature. The complex algorithms can produce results without providing a clear understanding of how those results were derived, making it difficult for chemists to trust and validate the outcomes.
Quantum-Aided Drug Design (QuADD) uses physics-based models that offer explainable outcomes. Chemists can understand the reasoning behind the outcomes, leading to higher confidence in the accuracy of the results.
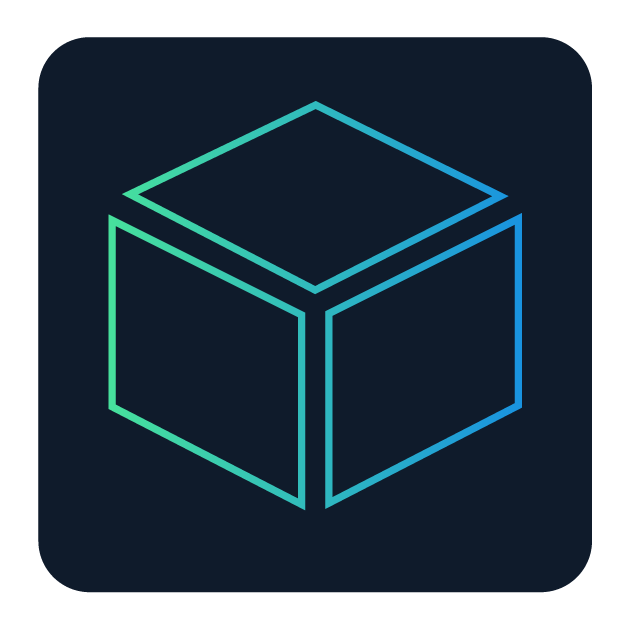
Quantum-Aided Drug Design (QuADD):
Leapfrogging AI Limitations
Targets limited by training data set
QuADD
Can be applied to any drug target with a known 3D structure
Why it matters
Even the latest AI solutions are limited to targets with extensive data for training, while quantum-based solutions can be used for a wide range of targets, including those that are novel or less studied.
Bottom line results
Apply to any target
Training data is often limited to thousands of compounds
QuADD
Can search a chemical space of 1030, the largest explorable today
Why it matters
By searching a space that is orders of magnitude larger, QuADD offers a greater likelihood of finding the ideal molecule compared to AI models that are limited in their ability to sample a vast chemical space.
Bottom line results
Discover hard-to-find molecules
Limited to training data diversity
QuADD
Can explore diverse chemical structures
Why it matters
AI’s reliance on training data means it may miss diverse and novel structures, whereas QuADD’s vast search space includes diverse chemical scaffolds, increasing the chances of discovering unique drug candidates.
Bottom line results
Generate a diverse candidate library
Black box
QuADD
Explainable physics-based model
Why it matters
Scientists can assess the candidates suggested by QuADD based on their physical interactions between the ligand and the pocket, whereas AI requires synthesis prior to understanding
Bottom line results
Minimize time and cost
Prone to generating unfeasible results
QuADD
Realistic results based on physical principles
Why it matters
AI models can sometimes generate chemically implausible compounds (“hallucinations”) or are ineffective due to overfitting or biases in the training data.
Bottom line results
Guarantee viable outcomes
Variability due to training data
QuADD
Consistent results based on quantum principles
Why it matters
QuADD provides reproducible results as it is not influenced by the variability of training data, ensuring consistent and reliable outcomes.
Bottom line results
Ensure reliable results